公共政策前沿研究方法导论
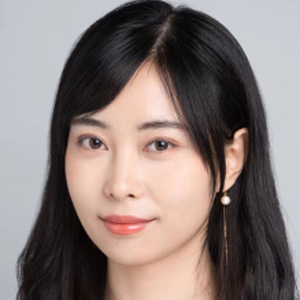
课程教师
Meijun Liu
教师简介
Meijun Liu serves as an Assistant Professor at the Institute for Global Public Policy, Fudan University. She earned her Doctorate degree in Information Science from the University of Hong Kong. Her research interests span across informetrics, science of science, and Science & Technology policy. Her work primarily revolves around three key themes: (1) international collaboration in science and technology, (2) the integration and diffusion of innovative knowledge through the use of cutting-edge Artificial Intelligence methodologies, and (3) the data-driven international mobility of scientists. She has been leading projects supported by the National Natural Science Foundation and Science and Technology Commission of Shanghai Municipality. Her research is published in high-quality international academic journals in information science, such as JASIST, Information Processing & Management, Journal of Informetrics, and Quantitative Science Studies. Her studies were featured on Nature and the Atlantic daily.
课程内容
This course offers an interactive exploration of advanced quantitative research methodologies in the realm of social sciences, with a particular emphasis on Public Policy. The curriculum encompasses the basics of research methods, causal inference, network analyses, decision-making methods, natural language processing including topic modeling and sentiment analyses.
The primary objective of this course is to equip students with a comprehensive understanding of cutting-edge research methodologies in Public Policy, facilitating the collection, analysis, and hypothesis testing of quantitative data. The course delves into the principles of scientific inquiry and a variety of research techniques.
Furthermore, we will introduce students to several research tools instrumental in conducting empirical analyses in Public Policy, including STATA, Gephi, and Python. This hands-on approach ensures that students gain practical experience and confidence in applying these techniques in real-world research scenarios.
预期目标
By the conclusion of this course, students are expected to have the ability to:
Craft pertinent research queries within the field of Public Policy and choose suitable methodologies to address them.
Cultivate quantitative research abilities which allow for the design and execution of independent empirical studies.
Utilize tools such as STATA, Gephi, and Python to carry out empirical analyses, employing the correct research methods.
课程安排
Lecture | Topic (2.5 teaching hours) |
1 | Couse description and basics of research methods |
2 | Causal inference: difference in differences |
3 | Casual inference: propensity score matching |
4 | Casual inference: instrumental variable |
5 | Network analyses: social networks—concepts, theories and application |
6 | Advanced decision-making method: decision tree and random forest |
7 | Natural language processing: topic modeling with BERTopic |
8 | Natural language processing: sentiment analysis |
9 | Applications of frontier research methods |
10 | Emerging trends and future directions |
Reading List
Babbie, E. R. (2020). Chapter 1: An Introduction to Inquiry. In The practice of social research: Cengage learning.
Knill, C., & Tosun, J. (2020). Chapter 1: Introduction. In Public policy: A new introduction: Bloomsbury Publishing
Babbie, E. R. (2020a). Chapter 5: Conceptualization, Operationalization, and Measurement. In The practice of social research: Cengage learning.
Angrist, J. D., & Pischke, J.-S. (2008). Chapter 5: Parallel Worlds: Fixed Effects, Differences-in-differences, and Panel Data. In Mostly Harmless Econometrics: An Empiricist's Companion: Princeton University Press.
Caliendo, M., & Kopeinig, S. (2008). Some practical guidance for the implementation of propensity score matching. Journal of economic surveys, 22(1), 31-72.
Angrist, J. D., & Pischke, J.-S. (2008). Chapter 4. Instrumental Variables in Action: Sometimes You Get What You Need. In Mostly Harmless Econometrics: An Empiricist's Companion: Princeton University Press.
Rashka, S., & Mirdzhalili, V. (2020). Chapter 10: Predicting Continuous Target Variables with Regression Analysis. In Machine Learning and Deep Learning with Python, scikit-learn, and TensorFlow 2. Birmingham, Mumbai. Packt.
Grootendorst, M. (2022). BERTopic: Neural topic modeling with a class-based TF-IDF procedure. arXiv preprint arXiv:2203.05794.
Rashka, S., & Mirdzhalili, V. (2020). Machine Learning and Deep Learning with Python, scikit-learn, and TensorFlow 2. Birmingham, Mumbai. Packt.
Student Assessment
The evaluation for this course will be distributed among: attendance (30%), active involvement in class (30%), and the final presentation (40%).
Regular attendance for every session is mandatory. Each unexcused absence or frequent tardiness will result in a 1% deduction from the final course grade. Active participation, which constitutes 30% of the final grade, is highly encouraged. This component will be assessed based on both the frequency and the quality of individual contributions to class discussions.
The course concludes in the 10th week with a 15-minute presentation by each student or group of students. This presentation should outline their research design, including the background of the research, identified gaps in the field, specific research questions in Public Policy that pique their interest, the application of pertinent data and research methods to address these questions, potential limitations, and anticipated results.